论坛时间:2022年06月01日(星期三)晚上20:00 (北京时间)
主 题:从单目深度估计到单目三维场景重建
主 持 人:刘昊(宁夏大学)
直播网址:https://live.bilibili.com/22300737
报告嘉宾:沈春华(浙江大学)
报告时间:2022年06月01日(星期三)晚上20:00 (北京时间)
报告题目:从单目深度估计到单目三维场景重建
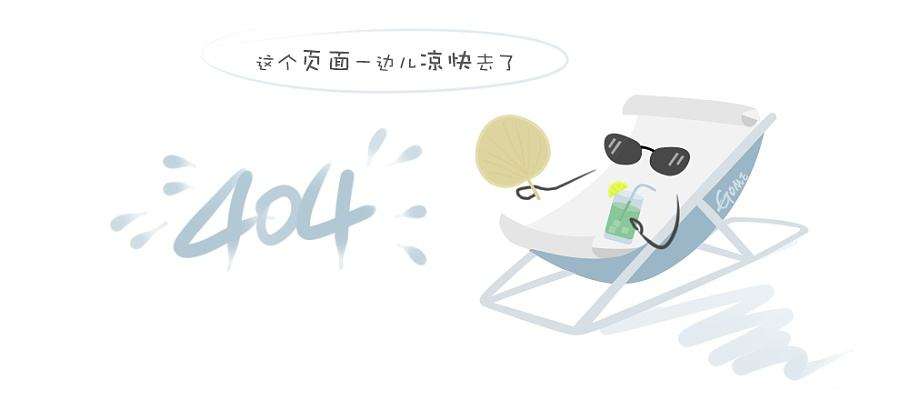
沈春华,浙江大学求是讲席教授。2021年底他全职加入浙江大学计算机学院、计算机辅助设计与图形学国家重点实验室。2011到2021年,他在澳大利亚阿德莱德大学计算机学院、澳大利亚机器学习研究院(australian institute for machine learning)、以及澳大利亚机器视觉卓越中心(australian research council centre of excellence for robotic vision)从事教学和科研工作;在这之前他在national ict australia堪培拉实验室以及澳洲国立大学工作了近6年。2012年获得australian research council future fellowship。 在从事教学的过去15年间,他指导毕业了28名博士生、30余名访问博士生。他在阿德莱德大学指导的博士生中超过一半学生毕业时获得了大学卓越博士论文研究生院院长奖(dean’s commendation for doctoral thesis excellence);4人获得google phd fellowship。他曾经担任澳大利亚monash大学数据科学及人工智能学科的客座教授、南京大学客座教授。他本科就读于南京大学强化部、南京大学电子系硕士、阿德莱德大学博士。他的研究兴趣主要在计算机视觉的几个基础任务,包括目标检测、语义分割、实例分割,单目深度估计以及3d场景重建等。他的google scholar引用达39000 ,h-index 100。
个人米乐体育官方下载主页:
报告摘要:
单目深度估计是计算机视觉的一个基础问题:在只有一张rgb图像作为输入的情况下,要求算法预测出每个像素点的深度(景深)值。单目深度估计在机器人导航、自动驾驶、增强现实、三维场景重建和理解等多个领域都具有广泛的应用。传统的深度估计方法,如slam,structure from motion等,都是建立在多个输入图像的特征点匹配上。数学上,从单个图像中推断深度信息是一个病态(ill posed)问题。2012年alexnet出现之后,利用大数据训练模型,对单个rgb图像进行端到端的稠密深度图估计取得了突破性的成果。我们从2014年开始研究基于神经网络的单目深度估计,为了提高估计的精度以及模型的泛化性,我们从训练数据的标注(labelled vs. unlabelled)、大规模训练数据的构建以及处理、在神经网络中引入先验知识等几个维度讨论这个问题,取得了一些阶段性的成果。在这个报告中,我将简要介绍一下我们过去8年在这个方向上做的几个代表性工作,并讨论有待解决的问题。
参考文献:
1. learning to recover 3d scene shape from a single image. w. yin, j. zhang, o. wang, s. niklaus, l. mai, s. chen, c. shen. proc. ieee conference on computer vision and pattern recognition (cvpr’21), 2021.
2.virtual normal: enforcing geometric constraints for accurate and robust depth prediction,w. yin, y. liu, c. shen. ieee transactions on pattern analysis and machine intelligence (tpami), 2022.
3.auto-rectify network for unsupervised indoor depth estimation,j. bian, h. zhan, n. wang, t. chin, c. shen, i. reid. ieee transactions on pattern analysis and machine intelligence (tpami), 2022.
4. unsupervised scale-consistent depth and ego-motion learning from monocular video,j. bian, z. li, n. wang, h. zhan, c. shen, m. cheng, i. reid. proc. advances in neural information processing systems (neurips’19), 2019.
5. monocular relative depth perception with web stereo data supervision,k. xian, c. shen, z. cao, h. lu, y. xiao, r. li, z. luo. proc. ieee conference on computer vision and pattern recognition (cvpr’18).
6. learning depth from single monocular images using deep convolutional neural fields, f. liu, c. shen, g. lin, i. reid. ieee transactions on pattern analysis and machine intelligence (tpami), 2016.
7. deep convolutional neural fields for depth estimation from a single image.f. liu, c. shen, g. lin. proc. ieee conference on computer vision and pattern recognition (cvpr’15), 2015.